Exploring Criminal Liability of Autonomous Systems
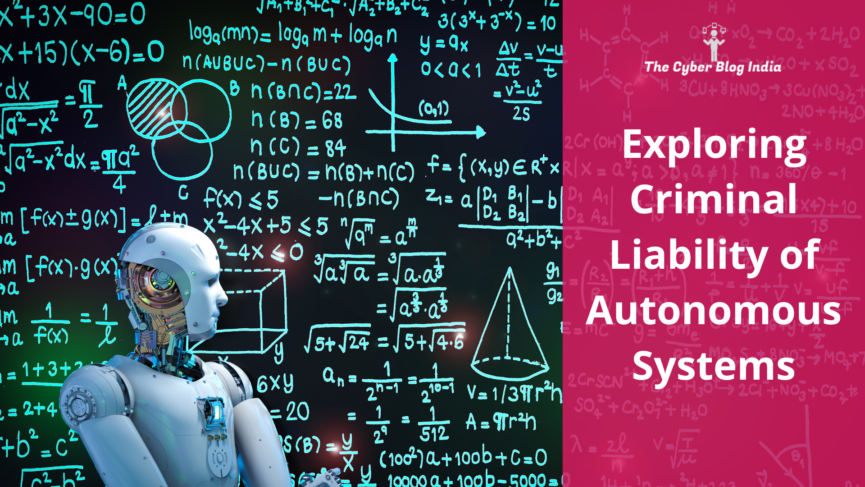
A sense of ‘right’ and ‘wrong’ is intrinsic to understanding law. For humans, this self-consciousness reflects our ability to judge whether our actions are moral and ethical. Researchers believe that AI can imbibe knowledge systems and make judgements accordingly. Some proponents of this theory claim that AI will soon become more human-like and surpass human intelligence. Thus, AI already has a sense of right and wrong and possesses a ‘rudimentary consciousness‘.
This engenders a discussion on the legal personhood of AI and whether these systems should be regarded as mere machines working at human command, autonomous entities that can think for themselves, or a mix of both. One section claims that AI systems have sensory receptors that analyse received data, comprehend it, and act accordingly. Thus, they are capable of actively interacting with people. Recognising the legal personhood of AI systems and allowing definitions of obligations and liability is essential.
The other section argues that AI is developed by people, and so AI systems are simply machines working at the behest of their human masters. This view was illustrated in a 2013 mock trial at the International Bar Association Conference. Here, the point of debate was whether or not an intelligent computer had the right to maintain its existence. The proceedings came to an anticlimactic end without a conclusion due to the lack of legislation governing the issue.
Conditions for Criminal Liability
To impose criminal liability on an autonomous system, one also needs to consider autonomous systems that may emerge in the future. In this context, we need to consider the following conditions.
1. Moral Algorithms
Most human choices are determined by what society thinks is morally right or wrong. Human beings value societal moral norms, which they internalise through social conditioning as they grow up. We can call such conditioning moral algorithms. However, moral algorithms assume a different context when it comes to autonomous systems. Autonomous systems that can make moral choices will have to have a moral algorithm in place, similar to the social conditioning of human beings. Available literature suggests two approaches to building moral algorithms in AI systems: rule-based and utility-maximisation approaches.
(a) Rule-based Approach
This approach involves coding moral rules that an autonomous system can apply when making moral choices. A strict rule-based approach requires coding all possible moral rules into the system. The developers must train the system on every moral dilemma that may potentially arise. Here, the system should not have room to use its autonomy in making choices. However, such a system can fail when an unprecedented ethical dilemma arises. Moreover, it will not be an autonomous system in the true sense of the word. Such a system will not use new learnings to execute its own decisions.
On the other hand, a soft rule-based approach encodes only high-level moral rules into the system (like templates). Further, it trains the system to demonstrate how those rules apply in certain moral situations. The system is then expected to learn independently and evolve its moral algorithm to address diverse moral dilemmas. In this way, the system is autonomous and consonant with general socially determined moral norms. One example of such a system is the Medical Ethics Expert (MedEthEx), which implements bioethical principles such as autonomy, nonmaleficence, beneficence, and justice.
(b) Utility-Maximisation Approach
This approach involves training the AI system morally using reinforcement learning. Here, when the system takes or executes a moral decision affecting its environment, the environment provides a critical evaluation of the system’s actions by giving numerical reward signals. The system will always strive to act in a way that maximises the total quantity of rewards. This method is similar to how humans learn in their childhood. If a child performs an act and gets a positive response from the immediate environment (parents, teachers, or friends), they know that they have performed a positive action and will again do it in the future. Conversely, they avoid performing the action again if they get a negative response.
In determining whether an autonomous system satisfies the condition of moral algorithms, one is essentially determining whether or not the system is capable of making moral decisions. A system that satisfies this condition is also more likely to inflict significant emotional harm on others. In case something goes wrong, one knows that the system has deliberately decided to cause harm to another. The harm no longer remains a technical fault but the system’s intention to do so. Attributing criminal liability to such a system is easier and has a rationale behind it.
2. Ability to Communicate Moral Decisions
Regardless of the approach adopted in designing and training a system on morality, it should be able to communicate its moral decisions to human beings. The system should communicate:
- The courses of action it will follow in a particular moral situation,
- How it weighed them out,
- What the priorities are with which it operates, and
- Why did it ultimately choose the course of action it did?.
For example, suppose a smart car crashes into a sidewalk. The vehicle must explain to a human being why it did so. Perhaps it had the option to crash into a human being crossing the street or the sidewalk. It chose the sidewalk, thus saving a human life through its decision-making.
The ability to communicate moral decisions is pertinent as humans are interested in determining who is responsible for a breach as and when it occurs. If a system cannot communicate its moral decisions, it becomes difficult to determine whether it has merely malfunctioned or made a deliberate moral decision. Determining its liability becomes difficult if it cannot provide the reasoning behind its actions. There can be numerous reasons why an autonomous system makes a particular decision.
3. No Immediate Human Intervention
The third condition is whether immediate human supervision is involved in the system’s decision-making processes. Under this condition, one assumes that the human being who receives instructions or ethical advice from the system follows them without questioning the intention behind them or applying their own judgment before acting on the instructions.
If a system has immediate human intervention, it means that there was human intervention in the final decision. In case of a breach, the liability will shift towards the human being who made the final moral decision.
Reasons for Criminal Liability
The question of whether autonomous systems should be held criminally liable depends on the type of personhood granted to them. Since this is an emerging area of research, there are views for and against imposing criminal liability on autonomous systems. The following sections elaborate on why this is essential.
1. Censure Wrongful Acts
The most apparent reason for imposing criminal liability on autonomous systems is to establish a mechanism for holding them responsible for their actions. Tort or civil law is more or less morally neutral. However, criminal law clearly conveys that a particular act is morally wrong. The person who committed it must bear the consequences. Before imposing criminal liability on AI systems, we must ensure they act independently without human intervention or system fault.
Penalising an autonomous system for a moral decision that injures a human being sends a clear message of the collective disapproval of its decision. This approach must consider all forms of autonomous systems, from smart cars and homes to fully autonomous weapons capable of mass destruction. In every scenario, criminal accountability for autonomous systems becomes paramount.
2. Mitigate Emotional Harm
People react emotionally to harm caused by non-human entities much like they would to harm caused by a human being. This is evident in how they react to a company’s wrongful actions and assign moral accountability. People are also known to have strong emotional attachments to objects and non-human entities despite being fully aware that they are not humans. A team of Japanese scientists found physiological evidence of humans empathising with robots that appear to be in pain. Studies have shown that people tend to blame robots for their wrongful actions. Their tendency to blame increases as robots become more human-like. Not allowing the victim to blame the autonomous system or not having an accountability mechanism will be insensitive and result in a trivialisation of the victim’s pain.
3. Deterrence and Other Values
Criminalising certain acts performed by autonomous systems may have a deterrent effect on them. They should take into account criminal law to regulate their own behaviour. Some might argue that instead of imposing criminal liability, we can programme autonomous systems to refrain from performing generally prohibited actions. However, criminal liability helps identify the individuals responsible for the harm caused. This can also encourage programmers to establish mechanisms to prevent wrongful conduct by the system.
However, there are some objections to imposing criminal liability on autonomous systems. The following section discusses them in detail.
Objections Against Imposing Criminal Liability
1. Autonomous Systems are not Agents
Action is done with intention instead of a natural happening or occurrence. Intention is personally determined. One may argue that an autonomous system is incapable of harbouring personal determinants. Therefore, intention is always absent. Hence, any action performed cannot be attributed to an autonomous system.
However, an autonomous system can act intentionally. Its moral algorithms function like that of an internal decision-making structure in a corporation. Therefore, any act the system performs with its moral algorithm for its own reasons can be deemed intentional. Sometimes, the coding of moral algorithms is so complex and constantly evolving that it is difficult to pinpoint the exact rationale behind an action performed by the system. In such situations, the system is the best authority on making its own decisions. Therefore, rather than attributing liability to anyone else, we must hold the system itself liable.
2. Incapability of Performing Morally Wrong Actions
An action is morally wrong if there is a moral rule or principle that categorises that particular action as wrong. According to the famous M’Naghten rules, the defence of insanity is only available to a person who does not know the nature and quality of the action they are performing. Therefore, to impose criminal liability on autonomous systems, we need evidence that the system is aware of a moral rule governing its action and that the rule proscribes the action.
Such evidence is difficult unless the system is specifically programmed to commit morally wrong actions. However, one also needs to consider that an autonomous system has moral algorithms designed by individuals who, one can assume, know the moral rules and principles of their society and are recognised members of their community. One can, in turn, recognise autonomous systems as members of the community and expect that they abide by moral principles. Consequently, if an autonomous system performs any action violating moral norms, one can impose sanctions on them and hold them criminally liable.
3. Responsibility for Actions
One can argue that an autonomous system is not responsible for its own actions because it did not choose the moral principles governing it. However, this implies that it is not truly autonomous. Here, the autonomy of a moral agent is considered in the Kantian sense. In this context, we must treat the agent as the author of its desires. If external factors engineer moral algorithms into an autonomous system, we cannot consider them truly autonomous.
However, alternative theories suggest that the strict application of Kantian autonomy is not necessary to deem a system autonomous. For example, List and Pettit provide three conditions in which we can attribute liability of its actions to an agent:
- The agent faces a normatively significant choice: whether to do something good or bad, right or wrong.
- The agent has the understanding and access to the evidence required to make normative judgments about the available action.
- The agent has the control required to choose among the available options.
Applying these three conditions to an autonomous system satisfies all of them. An autonomous system may often face ethical dilemmas where it must choose between good and bad. The system has moral algorithms to guide it in making normative decisions, satisfying the second condition. An autonomous system also has the control to choose its preferred course of action. In this manner, autonomous systems satisfy all three conditions of List and Pettit. This theory is just one example from the several proposed for this issue. Hence, it is safe to conclude that Kant’s theory of autonomy is not necessary to hold the systems responsible for their actions.
Models for Affixing Liability
According to traditional criminal law principles, actus reus and mens rea are two components of criminal law. Actus reus refers to the act of commission of an offence, and mens rea refers to the intention to commit an offence. Even if the former of the two is present in an autonomous system, the latter’s applicability is debatable. The intention to commit a crime requires the knowledge of its probable consequences. Researchers have developed various models; however, they all have attracted a fair share of criticism. We have discussed some of these models below.
1. Perpetration by Another Model
This model looks at autonomous systems as innocent agents. If the programmers determine their programming and learning methods, the intent is derived from their inputs. Hence, mens rea gets attributed to the programmer who programmed it in a way that led the system to commit the offence. Here, an autonomous system is simply understood as a tool its controller uses to perpetrate an offence.
It imposes strict liability on producers, as in the case of damages caused by manufacturing defects. EU’s Directive 85/374 also reflects this principle while focusing on manufacturing defects. The person(s) who produced the product are held accountable under the rule of strict liability, regardless of whether or not the conduct of the AI system was planned, intentional, or even foreseeable. This is comparable to vicarious liability, which holds an employer liable for the wrongdoings of an employee if they perform an action in the course of their employment. This draws attention to the fact that this situation is analogous to holding parents accountable for their children’s actions after the child has left their care.
Researchers have criticised this theory because it disregards AI’s independent decision-making capabilities while inhibiting innovation by imposing disproportionate burdens on producers.
2. Natural Probable Consequence Model
This model relies on autonomous system producers’ obligation to use reasonable care and avoid untoward incidents. The criterion for a negligence claim is simply avoiding any act or omission that a rational person would do to avoid harm. HM Roff, a proponent of this model, argues that any unfortunate incident caused by AI results from its creators’ failure to avoid risk and provide adequate warning.
However, the standards of reasonable care are vague and undefined. There are no international legal norms to help define these in the context of AI systems. Even if reasonable care is taken, the manufacturer cannot determine the course of action of the systems as they do not have the skills to do so. This becomes particularly problematic in the case of advanced AI systems that learn from environmental data and adapt to their surroundings. Creators have no feasible way to predict their behaviour. Some argue that holding creators to such a high standard would be unjust. Again, the blanket liability of the manufacturer would also stifle innovation and creativity.
3. Direct Liability Model
This module assumes that AI does not work at the behest of any person and makes decisions independently. Exponents of this model believe that the only legal requirements needed to affix liability for a crime are knowledge and specific intent, both of which autonomous systems possess. Recognising the autonomy of systems is vital to holding them liable.
Dissenters claim this theory is flawed as it generalises diverse forms of AI, assuming that all autonomous systems can make independent decisions. In reality, AI systems’ cognitive development levels vary widely, and holding them all liable is erroneous. Dias talked about how blame can only be attributed to a free being. Based on this, an offender is declared culpable on the assumption that they had a choice to commit or not to commit an offence. AI systems often are not capable of the same. Thus, they cannot have legal rights or be held liable.
Conclusion
The question of AI’s legal personhood and criminal liability remains complex and multifaceted. While AI systems can analyse data, learn, and make decisions, attributing human-like consciousness or moral agency is premature. The debate hinges on whether AI is a mere tool or an independent entity with its own moral agency. Establishing criminal liability requires careful consideration. Conditions like the presence of moral algorithms, the ability to communicate moral reasoning, and minimal human intervention are crucial. However, challenges remain. Moreover, objections arise from the perceived lack of intentionality, the difficulty of proving moral awareness, and the question of true autonomy in AI systems.
Proposed models for affixing liability, such as the perpetration by another model, the natural probable consequence model, and the direct liability model, each present advantages and drawbacks. The perpetration by another model focuses on the creators’ responsibility, while the natural probable consequence model emphasises the duty of care. While the direct liability model recognises AI’s potential autonomy, it raises concerns about holding systems without full moral agency accountable.
A nuanced approach is necessary moving forward. Continued research into AI ethics, the development of robust legal frameworks, and open dialogue between legal scholars, technologists, and ethicists are crucial. As AI technology evolves, so must our understanding of its legal and ethical implications. Ultimately, a balanced approach is needed, recognising AI’s potential benefits while mitigating risks and ensuring responsible development and deployment.
Akshita Rohatgi, an undergraduate student at the University School of Law and Legal Studies, GGSIPU, and Shrawani Mohani, an undergraduate student at ILS Law College, Pune, worked on this research piece during their internship with The Cyber Blog India in January/February 2021.